Monitoring Asset Health with Megha AI Solution.
- meghaai
- Jul 10, 2022
- 4 min read
Updated: Jan 11, 2023
Industry 4.0
The Fourth Industrial Revolution, or Industry 4.0, is bringing rapid changes within the industry from the evolution of technology, largely due to increasing interconnectivity, smart automation, and modern technologies like artificial intelligence, big data, and machine learning and building technology to derive insights from data and technical processes.
This movement is making fundamental shifts toward the “Smart Factory” through automation of existing manufacturing and industrial practices using the internet of things (IoT). This adoption of smart sensors and IoT is resulting in increased automation, improving communication between machines, between processes, between machines and processes, between people and machines/processes, and self-monitoring, as well as the use of smart machines that can analyze and diagnose issues without the need for human intervention. The applications of Industry 4.0 range in wide technologies like sensors, the Internet of Things, Mobile, Cloud Computing, Big Data, AR/VR, AI-ML, and many more.
Industry 4.0 and the “Smart Factory” is no longer a distant goal. Various elements within manufacturing: people, process, product, quality, performance, and machine metrics can all exchange data with each other with IoT and other high-speed communication channels like 5G and efficient protocols like MQTT. The concept of the “digital twin” and its reality through the cloud services like AWS IoT Site Wise, and AWS digital TwinMaker, can bring the system components of the factory to the centralized technological platform for better insights and data-driven decision making.
Megha AI through the I4.0 Accelerator solution powered by AWS can gather data from assets and energy reading systems to optimize energy efficiency using AI/data analytics. This solution is projected to drive down energy costs between 8-20% depending on the factory’s current the efficiency of operations.
Megha AI also provides an Asset Health solution. This is an anomaly detection platform that scans the health of machines, alerts when there is abnormal behavior, and provides a root cause analysis as to where the anomaly occurred. These analytics help industries move towards predictive maintenance and allow for plant owners to determine when to schedule maintenance before machine failure and optimize cost.
This solution is projected to reduce product development costs by up to 50% and increase gross margin by 33%.
Solution Overview
Machine maintenance is the process of assessing, upgrading, and performing upkeep on machinery to ensure mechanical assets stay running with minimal downtime. Scheduling machine maintenance can be challenging for manufacturers today. Maintaining too frequently is waste of time and resources, whereas not maintaining machines until the point of failure can lead to substantial repair costs, delayed production schedules, and workplace accidents. Megha AI proposes a solution to machine maintenance scheduling wherein continuous monitoring of machines is applied using advanced machine learning and analysis which alerts the end-user when machines are behaving abnormally.
Megha AI RAIN (Rapid Actionable Insights Navigator) provides a digital twin of the plant floor, machine insights visualization, and machine learning models to monitor asset health.
Asset health functionality works with multiple related assets and associated measurements/tags and is not limited to a single asset.
Data Pipeline
The following block diagram summarizes the data flow from the factory to the visualization. Data from the industrial assets are captured and organized at the edge and sent to Amazon Web Services (AWS) Cloud to be stored, processed, and visualized.

Megha AI auto-extracts the data schema from the edge and transforms streaming asset values for data visualization, model training, and inference. This data pipeline is built under our intuitive, no-code user interface which provides uses a way to derive value from data insights without any prior knowledge of data science knowledge.

Business Benefits
Most manufacturers today operate with outdated, stale, data or no data at all. When data connectivity is established, there is an overwhelming amount of data published at a very high velocity. Without AI-ML tools for data analytics and Insights, it is impossible to digest and understand the millions of metrics published by machines.
Megha AI’s Asset Health is a breakthrough in the democratization of AI and ML technology- any manufacturer, plant operator, supervisor, or machinist can derive insights without the need for a data science organization. Asset Health facilitates development by providing user-friendly tools, pre-built algorithms, and intuitive interfaces within a high-performance cloud computing platform.
With Asset Health, end users can build AI models to identify and understand abnormal behavior on machines. Asset Health monitors machine parameters and alerts when abnormal behavior is detected. This technology increases assets uptime, performance, and quality of the parts being produced, and ultimately kick starts predictive maintenance across industries.
Solution Details
Asset Health provides a platform for end-users to build AI models to monitor the running behavior of assets and schedule an inference, which runs the model periodically in real-time, streaming data to assess for anomalous behavior.
The user can build new Asset Health models by selecting assets and their associated measurements/tags, and optionally loading past failures (timestamps). Models can support as many as 300 measurements.
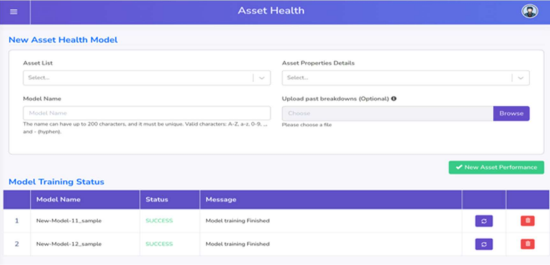
Once the Asset Health model is created, real-time streaming data from the assets can be scheduled for inference at the desired time intervals and frequencies. For example, critical equipment is monitored at a high frequency whereas less operational equipment does not need the same level of inference.
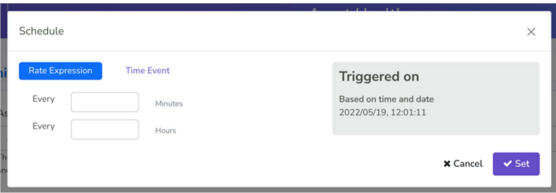
Asset Health allows users to understand the behavior of their machines over time by visualizing machine health and plotting sensor contributions to each abnormal asset event. Alarms can also be set on these results to notify users of critical metrics and potential machine failure.
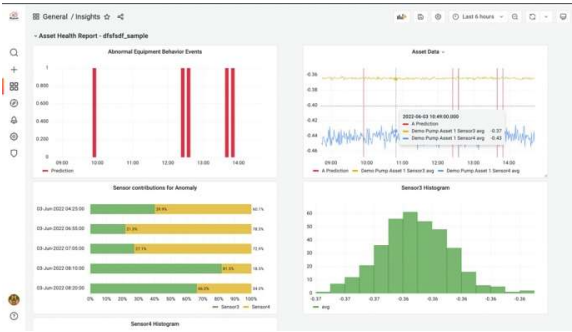
About the Authors
Krishan Kumar Meghani is an experienced software professional with 25+ years of software product development experience in the enterprise and Industrial suite of products, mainly in the oil, gas, and marine industries. He has led various Innovative development Products throughout his career for the success of the customers. He has six patents filed in his name along with co-inventors in machine learning and data science for industrial domains.
Aarthi Janakiraman is a data analytics and cloud industry professional for Megha AI. She currently works with several manufacturers across Indiana and helps define data science strategy and AI/ML execution within the industry 4.0 space.
Contact us
1-833-634-4281
support@meghaai.com
Visit us at
https://www.meghaai.com/
https://www.linkedin.com/company/meghaai/
Comments